To complement our series of QuickReads covering measurement, we will now explore the various data…
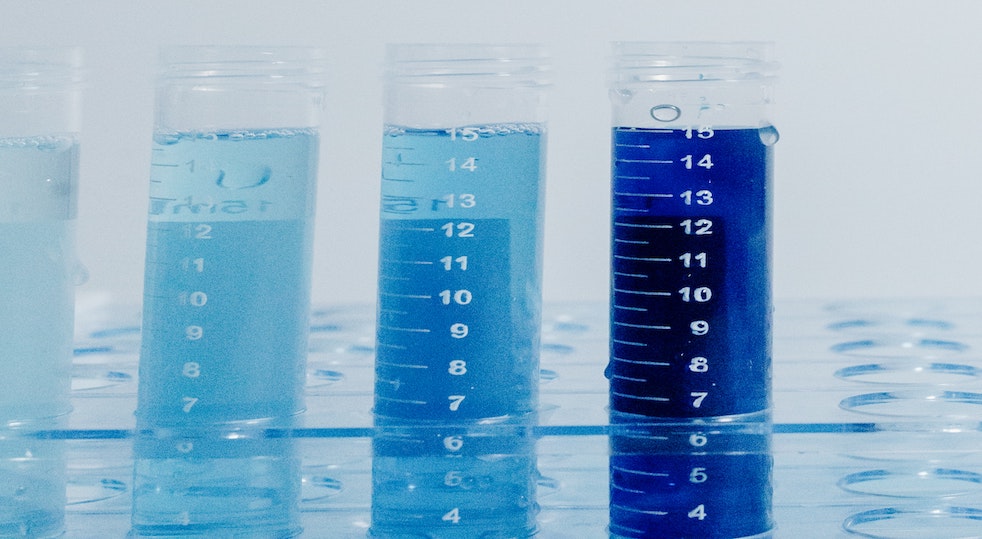
ChangeWise Quick Read: Measurement Series – Sampling Techniques
Continuing with our helpful series of QuickReads looking at measurement, we will now explore sampling with a focus on when to use it and the different types to consider when collecting data for your Lean improvement. Consider this session a quick recap of a school Geography lesson! 📚
What is Sampling in Lean?
Sampling means exactly what it says on the tin – collecting only a representative selection of the data available. The sample is then used to draw conclusions regarding the total data set.
You need to consider if all the data is required to make an informed decision at the start of your measurement phase.
But why? Surely it makes sense to collect all of the data? Then I can be certain I haven’t missed anything.
Not necessarily. Collecting all the data is often too expensive, too complicated – or simply non-value adding. Sampling is perfectly acceptable for understanding the ‘as is’ so long as the sample is representative of the full data set, mitigates variation, and takes into account all cost implications. In short, no systematic differences should exist between the data you collect and the data you don’t collect.
Ah, I see……..tell me more
Great, let’s get a bit technical and introduce some jargon!
There are four main types, let’s look at each in turn:
Simple Random Sampling
This type of sample is a subset of data chosen at random from a larger set of data. Each data item has an equal probability of being chosen so that the selection is unbiased. The data can them be used to make generalisations about the entire data set.
For example, I might want to find out if employees feel ChangeWise offers employees good opportunities to socialise with colleagues after work. Using simple random sampling I could select 25 employee names at random (out of a hat), rather than ask the total number of 100 employees to complete a questionnaire answering ‘yes’ or ‘no’.
If 20/25 employees answer ‘yes’ to the question ‘Do you think ChangeWise offers good opportunities for employees to socialise with colleagues after work?’, I can draw the conclusion that the majority of employees feel the same. Based on the percentages from my sample, it is likely a survey of all employees would result in 80/100 answering ‘yes’.
Simple Random Sampling is generally a quicker and less expensive method for drawing conclusions. However, the major disadvantage is that it can never fully guarantee accurate representation of the whole. This is regardless of random selection and especially if you access to the complete data set is not available.
Stratified Random Sampling
This method involves sampling from a full data set which can be partitioned into data subsets. Samples are randomly extracted from each subset to ensure the entire data set is represented fairly.
Using the above example, I might divide ChangeWise employees into subsets by department and take random samples from each subset.
Whist this method ensures one single data subset does not misrepresent results, arranging and evaluating the results can be more complicated.
Systematic Random Sampling
This method is the same as random sampling but with a fixed periodic interval. This is a great way to include representation for a large set of data and provided there is no opportunity for data manipulation, this method can work well in representing the full data set.
If using this method, for my survey example, I might select every 5th ChangeWise employee from a full list of employees.
If using this method, be careful to make sure you don’t under or overrepresent particular patterns. For example, if sampling every 100th widget on a production line for quality issues, this method would be unsuitable if there were any changes to production/machinery in proximity to every 100th widget.
⚠️ Important Point! Are your measurement gauges repeatable and reliable (Gauge R&R)? Before collecting the first data point, it is imperative to ensure that the measurement systems and gages are capable or providing information that represents the true process.
Subgroup Sampling
This sampling process involves taking a planned number of consecutive samples during each defined time period. For example, if a widget assembly machine produces sixteen glass parts per hour, I might use the subgroup sampling method by measuring three randomly selected parts every hour. In this scenario, each sample of three parts is a subgroup.
This method tends to work well provided you have a big enough sample/data set to work with. Issues occur if the data set is too small, or the subset selection is too small.
🏆 Top Tip!! A Measurement System Analysis (MSA) may be conducted to validate and ensure your measurement system is capable of collecting the data defined in the plan.
In Summary
Sampling is a great way to accurately answer questions about your process, it provides an easier method for data collection, it’s cost-effective and manageable. There are four main types of sampling and which one you select will depend on many factors including data size, variability, potential dependencies and outside influences on your data collection.
Looking for more information? Get in touch with the ChangeWise team at info@changewise.co.uk
ChangeWise believes employee engagement is the foundation for successful Change. Training and coaching your people to use simple continuous improvement techniques will enable your organisation to continuously adapt and stay ahead in a constantly changing and challenging environment.
For updates and interesting Lean Change insights, connect with us on LinkedIn.